In today’s data-driven landscape, the imperative for enterprises to leverage the potential of data while maintaining privacy and confidentiality has never been more pronounced. As organizations strive to strike a balance between collaboration and protecting sensitive information, innovative solutions emerge to meet these evolving needs. Enter the concept of data clean rooms – a practical solution that provides a privacy-protected environment where multiple parties can collaborate securely with their data assets.
This blog will delve into:
- The meaning of a data clean room
- Difference between traditional and distributed data clean room
- Applications of data clean rooms
- Distributed data clean room powered by Snowflake
What is a data clean room?
A data clean room is defined as a sophisticated and secure environment designed for collaborative data analysis while prioritizing privacy and confidentiality.
You can think of a data clean room as a virtual laboratory where multiple parties can come together to work with sensitive data without exposing individual privacy or propriety information. Let’s try to understand this with the help of an example. Let’s say there are two pharmaceutical companies ‘X’ and ‘Y’. They are considering a collaboration to develop a treatment for a rare disease. Both companies have valuable research data, including clinical trial results, genetic profiles, and drug efficacy risks. However, sharing all this information directly will pose significant privacy risks. This is where a data clean room comes in.
Both companies can establish a virtual workspace equipped with encryption protocols, access controls, and anonymization techniques. Within this secure environment, researchers from both companies can access and analyze the combined dataset without ever seeing identifiable information about individual patients or proprietary details about each other’s research. Researchers can explore correlations, identify patterns, and derive insights that may lead to groundbreaking discoveries—all while ensuring compliance with privacy regulations and maintaining the confidentiality of sensitive information.
“Data clean rooms provide a security-enhanced environment in which multiple parties can share, join, and analyze their data assets without moving or revealing the underlying data.”
Traditional Data Clean Room v/s Distributed Data Clean Room
Traditional data clean rooms and distributed data clean rooms are both environments designed to facilitate the processing and analysis of sensitive data while preserving privacy and confidentiality. However, they differ in their architecture and approach to data handling.
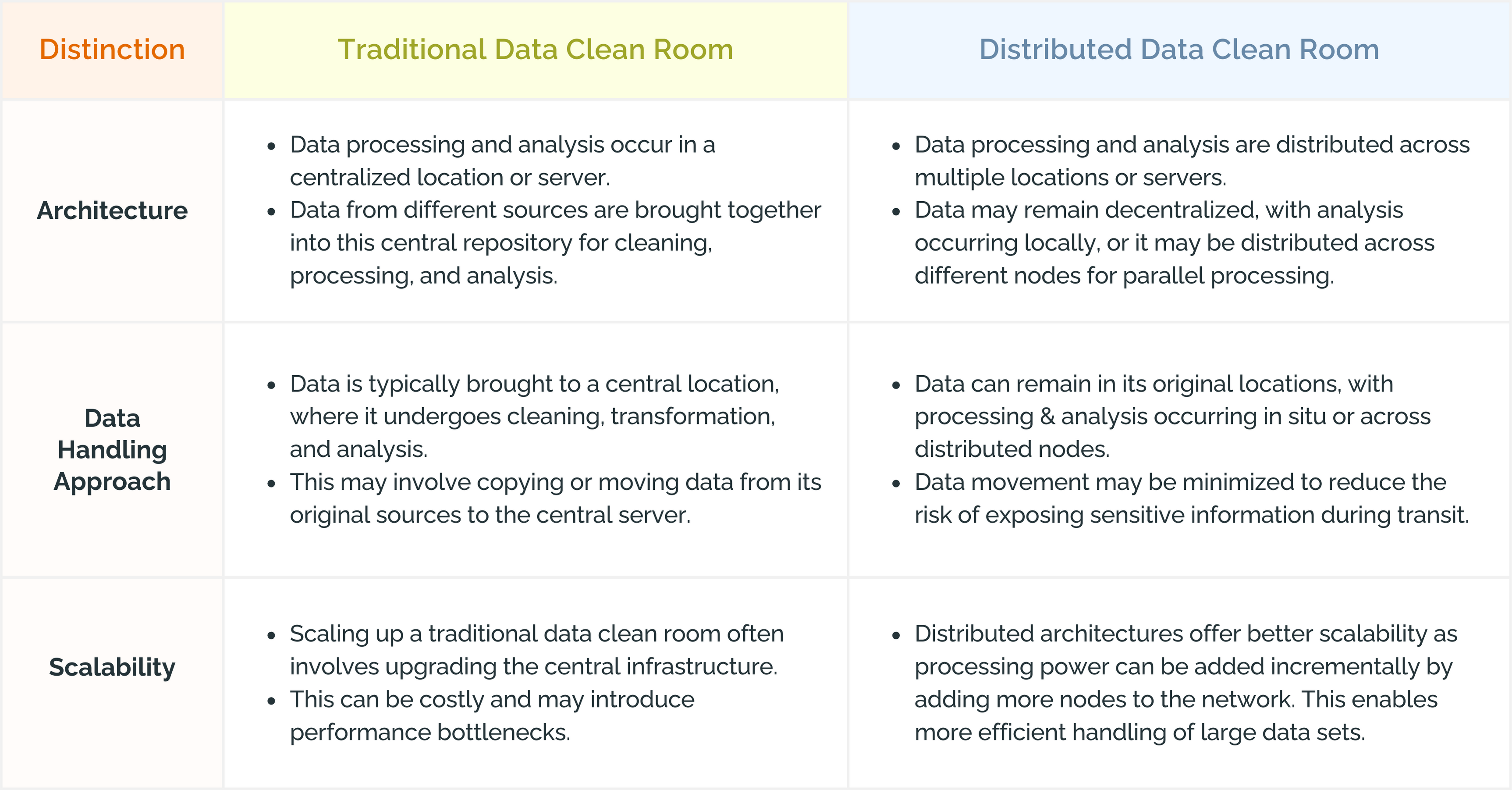
Traditional Data Clean Room vs Distributed Data Clean Room
Use Cases for Data Clean Rooms
Traditional data clean rooms and distributed data clean rooms are both environments designed to facilitate the processing and analysis of sensitive data while preserving privacy and confidentiality. However, they differ in their architecture and approach to data handling.
1. Customer Analytics and Marketing
- Retailers and e-commerce companies seek to analyze customer behavior, personalize marketing campaigns, and optimize inventory management while protecting consumer privacy and complying with regulations.
- Data clean rooms enable retailers to aggregate and analyze customer data from multiple sources, such as online transactions, loyalty programs, and social media interactions, in a secure and compliant environment.
- By applying advanced analytics techniques like segmentation, clustering, and predictive modeling within the clean room, retailers can gain insights into consumer preferences, identify purchasing patterns, and deliver personalized marketing experiences.
2. Financial Risk Assessment and Fraud Detection
As per PwC’s Global Economic Crime and Fraud Survey 2022, approximately half of the surveyed organizations reported experiencing instances of fraud.
- Data clean rooms enable financial organizations to aggregate and analyze data from various sources, including transaction records, market data, and customer information, in a secure and compliant manner.
- Advanced analytics techniques such as machine learning and anomaly detection can be applied within the clean room environment to identify fraudulent activities and assess risk factors.
- For instance, a bank could use a distributed data clean room to analyze transaction patterns across its customer base to detect unusual spending behavior indicative of fraudulent activities, without compromising individual account information or violating privacy regulations.
3. Healthcare Research
- Healthcare institutions often possess vast amounts of patient data scattered across different systems and databases. Data clean rooms can facilitate secure collaboration and analysis of this data for medical research, treatment optimization, and population health management.
- Researchers can access aggregated, anonymized patient records within the clean room environment. This enables them to identify patterns, assess treatment effectiveness, and develop predictive models for disease prevention.
Powering Data Clean Rooms with Snowflake
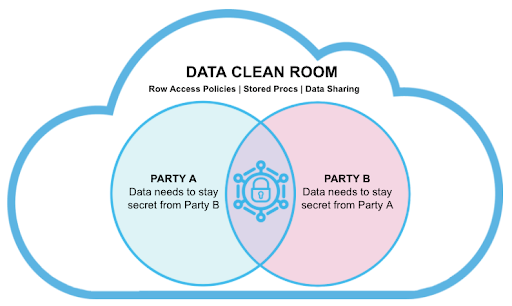
Image Source: Snowflake
Many organizations have long sought ways to collaborate securely on data without compromising individual privacy or exposing sensitive information. While secure data sharing has been a significant advancement in this regard, data clean rooms represent the next evolution in secure data collaboration methods.
In essence, data clean rooms provide a heightened level of security and control over data access and usage compared to traditional data sharing methods. With data clean rooms, organizations can define strict rules and policies governing the types of queries and analyses that can be performed on the data without granting direct access to the underlying data itself.
Furthermore, data clean rooms represent a more sophisticated approach to data collaboration, incorporating advanced security features, access controls, and auditing mechanisms to enforce compliance with privacy regulations and industry standards.
Why Snowflake?
Snowflake’s Data Clean Room functionality provides a powerful solution for sharing data without revealing sensitive information. This capability is achieved through a combination of advanced security features, access controls, and data masking techniques. Snowflake allows organizations to define access policies and permissions that restrict access to specific subsets of data or limit the types of queries that can be executed. This means that users or collaborating parties can only access the data they are authorized to view.
Here's How It Works
Suppose two companies that want to collaborate on data analysis. Each company can identify the specific datasets they want to share for joint analysis, without moving the data out of their respective Snowflake database accounts. They can “list” these datasets in a secure location within Snowflake where only authorized parties can access them. Once the datasets are listed, each company can configure access controls and apply secure functions to protect the data from unauthorized access or misuse. This includes setting permissions on who can view, modify, or analyze the data, ensuring that only the right parties have access to the information they need.
If both parties already have Snowflake accounts, they can immediately begin joint data analysis within the secure environment provided by Snowflake. Alternatively, if one party does not have a Snowflake account, the Snowflake customer can set up a secure subaccount for them, ensuring that they can still participate in the collaboration while adhering to strict security protocols.
A Manufacturing Company Example
Let’s say there’s a multinational automotive manufacturer that collaborates with a third-party advertising agency to launch a marketing campaign for its latest electric vehicle model. The manufacturer wants to target potential customers who are environmentally conscious and have shown interest in electric vehicles, without compromising individual privacy or exposing sensitive customer data. The automotive manufacturer possesses data on its existing customer base, including vehicle purchase history, demographic information, and preferences for eco-friendly features.
Meanwhile, the advertising agency has access to anonymized data from online forums, social media platforms, and automotive industry publications. This means they have insights into consumer sentiment, trends, and purchase intent related to electric vehicles.
Using Snowflake, the automotive manufacturer and the advertising agency can establish a data clean room to securely aggregate and analyze their respective datasets without sharing identifiable customer information. They can define access policies and allowed statements to ensure that only aggregated, anonymized data relevant to the marketing campaign is shared between parties.
Within the data clean room environment, the manufacturer and the agency can collaborate on identifying target audience segments based on shared characteristics. By applying advanced analytics techniques, such as machine learning algorithms and predictive modeling, they can identify potential customers who are most likely to be interested in the new EV model and tailor marketing messages
Wrapping Up
LumenData stands as a premier partner with Snowflake, offering unparalleled expertise in leveraging the platform’s capabilities. Reach out to discover our tailored accelerators and quickstart programs designed specifically for Snowflake, empowering your organization with rapid deployment and maximized value from your data initiatives.
Reference Links:
Authors
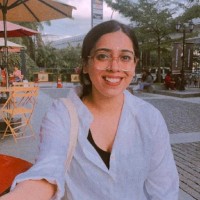
Shalu Santvana
Content Crafter
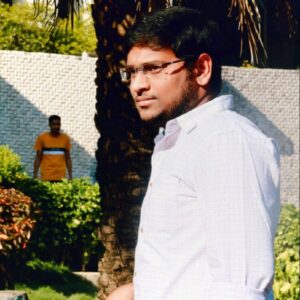
Sai Bharadwaja
Senior Consultant