You rely on vast amounts of information to make critical business decisions, identify the latest trends, and gain a competitive edge. However, the linchpin to unlocking the full potential of this data lies in ensuring its impeccable quality. Emphasis on data quality should not just increase; it should be at the forefront of every organization’s data strategy. Hence, businesses should proactively seek modern solutions to bolster it. Enter Reltio – a leading market player in cloud MDM solutions and your key to superior data quality.
In this blog piece, we’ll go over:
- Meaning of data quality
- Elements of data quality
- Reltio’s powerful capabilities to deliver trusted data
What is data quality?
Simply put, data quality refers to how well data serves its intended purpose or how effectively it meets the specific needs and requirements for which it was collected or leveraged. When data fits its intended purpose perfectly, it’s high-quality data. When data doesn’t reflect the real state of business affairs, it is low-quality/bad data and can lead to misguided strategies, investments, and operational decisions. It can also result in high IT maintenance costs. Research says that every year, organizations incur an average of $12.9 million due to poor data quality.
When your data reaches a certain quality standard, it instills confidence among stakeholders who rely on it. This trust in data allows businesses to effectively utilize it for enhancing decision-making. Trustworthy data supports fresh business strategies and helps refine the current ones.
Reltio defines data quality as “the central pillar in any data management framework, which assumes data is inherently dirty and challenged by multiple inequalities: incompleteness, inaccuracies, duplications, inconsistencies, invalidity, reasonability, and currency.”
Elements of data quality
Data quality encompasses several key components that together determine the overall reliability and usefulness of data. Let’s take a quick look at some of them:
1. Accuracy
It refers to the degree to which data correctly represents real-world scenarios or events it is supposed to describe. Accurate data is free from errors, inconsistencies, and discrepancies and provides a reliable representation of the information it is meant to convey.
2. Consistency
Consistency means the uniformity and coherence of data across an organization’s various systems and databases. Data values and formats are standardized and aligned, regardless of where and how the data is stored or accessed.
3. Uniqueness
Uniqueness is the degree to which data records or values within a dataset are distinct and free from duplicates. When data is not unique, it can lead it errors and incorrect conclusions. Imagine a customer database having the same customer’s information appear multiple times due to duplicate entries. It can result in inaccurate customer counts and repetitive marketing campaigns. Therefore, maintaining uniqueness in data is critical for data quality.
4. Timeliness
Timeliness means that data records are up-to-date, reflecting the most recent data available. Timely data ensures that stakeholders are working with the latest insights. This data quality element is crucial in dynamic environments/industries like healthcare, where changes can have significant implications.
5. Completeness
Completeness is described as the extent to which all required data attributes or fields are present and populated with information in a dataset. The data is whole and comprehensive, with no missing values or gaps.
6. Validity
Validity refers to the degree to which data conforms to predefined rules, standards, or constraints. Valid data will stick to the expected format, structure, and criteria set by the organization or industry standards.
Elevating data quality with Reltio
According to Forrester Total Economic Impact Study, customers leveraging Reltio achieved $4.7 million in IT cost savings. The Reltio Connected Data Platform empowers your data with unification, reliability, and real-time access for everyone. It is an ideal choice for enhancing data quality as it combines master data management capabilities with real-time data access and reliability. With its cloud-native architecture, it’s well prepared for the increasing shift towards cloud adoption.
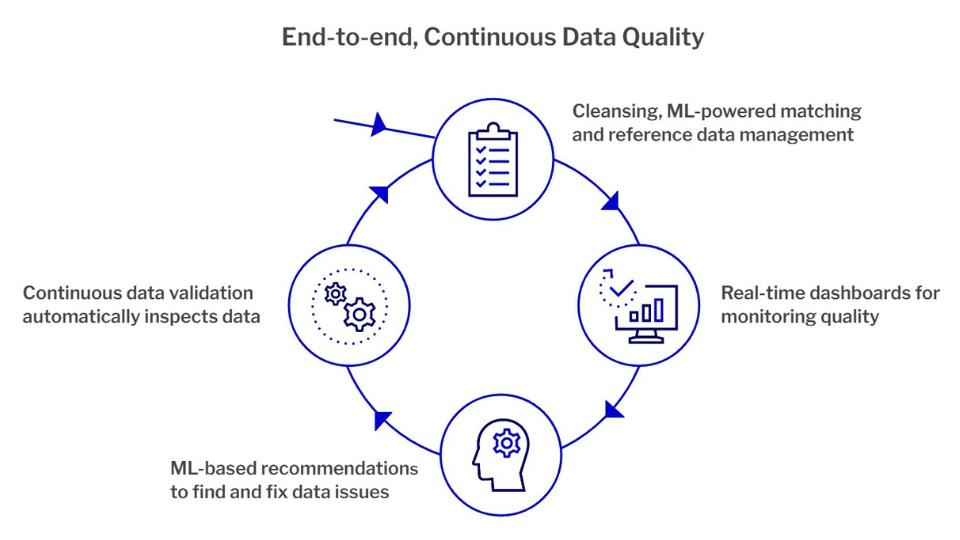
Image Source: Reltio
With Reltio, you get industry-leading data quality management that is continuous and automated. Here are some advantages you’ll gain:
• Enable continuous data validation
Continuous validation automates the process of detecting and rectifying data issues and reducing the risk of data degradation. It is a proactive approach to save time and resources and helps organizations maintain data integrity. Reltio’s powerful platform allows businesses to examine data efficiently and consistently through customizable business rules. You can promptly detect and highlight data irregularities, allowing for swift corrective actions.
• Facilitate quick quality fixes with ML-powered dashboards
When data quality problems are left unattended, they can lead to misinformation, operational inefficiencies, compliance violations, and even damage customer relationships. The “easy-touse” and “fully configurable” dashboards provided by Reltio are user-friendly interfaces that offer a real-time view of your data. You can view the state of your data as it exists right now, rather than relying on potentially outdated reports. Moreover, you can quickly spot anomalies that may be affecting your data. Reltio dashboards are customizable – you can tailor them to your specific needs and preferences.
• Conduct automated data cleaning
Reltio can receive data from multiple sources such as databases, applications, or external feeds. When data is ingested from various sources into the Reltio platform, it undergoes an automated and continuous process of cleaning and standardization. Common cleansing activities include removing duplicate records, correcting misspellings, and ensuring data formats are consistent. Data is standardized by converting the information into a uniform structure. This makes the data ready for consumption by downstream systems that may include business intelligence tools, analytics platforms, CRM systems, and more.
• Manage reference data to effectively govern sensitive information
Reference data, also known as static data, represents fixed values or categories that are used to classify or describe other data elements. Reltio’s platform allows organizations to define and maintain reference data sets related to products, customers, suppliers, and other relevant entities. Reference data is created, edited, and managed through a user-friendly interface, ensuring that it is accurate across systems. Reltio’s reference data management is adaptable to different domains and industries. It accommodates geographical variations in data, making it convenient for businesses operating in multiple regions with different data requirements.
Wrapping up
As trusted Reltio partners, we take immense pride in our extensive experience in modern data management. Our expert consultants closely collaborate with businesses to customize Reltio’s functionalities, aligning them seamlessly with unique company needs.
A leading pharmaceutical manufacturing company faced several challenges like lack of synchronized data, poor data quality, and inconsistent data across departments. LumenData helped the organization achieve data modernization with Reltio MDM deployment. We provided them with the following solutions:
• Real-time implementation of data sources.
• Formalization of data quality rules.
• Improved data stewardship.
Initiate a conversation with us to discuss how we can transform your data journey.
References
Authors:
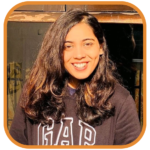
Shalu Santvana
Content Crafter
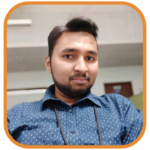
Mohd Imran
Senior Consultant
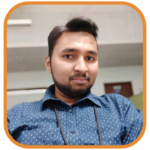
Mohd Imran
Senior Consultant
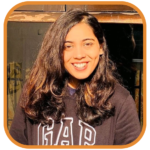
Shalu Santvana
Content Crafter